Role of AI and ML in the Automotive Sector: A roadmap for 2022
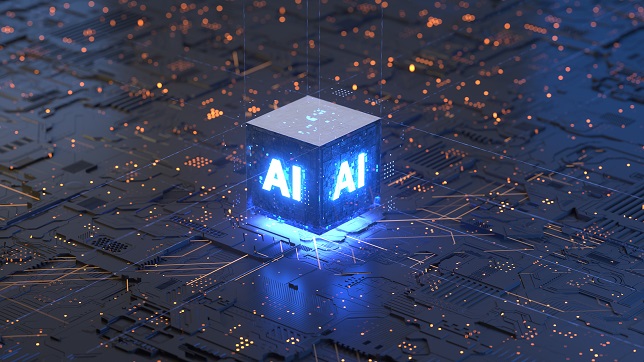
With the pandemic leaving a trail of disruption in its wake, the global automotive industry has been quick to adapt and evolve. The most prominent of these transitions is undoubtedly the growing relevance of AI and ML in the automobile sector. With industries around the world pivoting towards digital innovation, the automotive industry is one of the few sectors that is leading this transformation by harnessing these new age tech advancements. Let’s see what role AI and ML are slated to play in the automobile industry in 2022:
With Artificial Intelligence and Machine Language taking center stage across numerous arenas in the automobile industry, the most prominent perhaps is the EV sector. In stark contrast to the traditional IC powered engines that are a product of 120 years of research and development, the EV is still at an evolutionary stage and is yet to be fully embraced across mainstream vehicle platforms, especially in a country like India. However, as concerns for planet-friendly and emission free transportation grow deeper, the focus on EVs as the next automotive frontier is poised to increase.
And at the heart of the EV revolution is the optimization of AI and ML to enhance efficiency and effectiveness where it matters MOST. When it comes to EVs, the most crucial aspect is battery management, hands down. As the single most critical component of electric vehicles, the EV battery is responsible for dictating the overall shelf life of the vehicle. Therefore, EV players are laying deep emphasis on utilizing AI and ML for various battery management metrics like range prediction to gain accurate statistics on effective mileage achievable and health monitoring modules which can gauge the remaining useful life of the battery.
When it comes to EVs, battery management facets cannot be compromised. It is important that EV users gain a clear picture of battery performance beforehand to avoid unnecessary hindrances on account of the battery running out. Here AI and ML assay a huge role in determining the battery performance which depends upon a number of variables such as acceleration patterns, deceleration patterns, transmission utilization trends, and use of headlights and HVAC. The AI models need to contextually track the various behavioral patterns that take a toll on the battery. This includes being intuitive of subtle trends in operating modes which can affect the current output of the battery, such as the exact time when the lights are switched on. The AI and Ml-based predictive system must also include various ambient factors like temperature conditions and humidity levels which can impact the overarching performance of the vehicle.
In case of impending battery heating or leakage issues, these next-gen systems can profile factors which lead to chemical discharges and alterations prior to such events. Therefore Machine Learning systems must predict a plethora of both intrinsic and extrinsic factors to precisely estimate battery longevity. A number of deep tech firms are innovating various cutting-edge algorithms to assert accurate range-prediction for heavy duty vehicles. AI and ML based systems are also being utilized for quantifying performance metrics of emergent EV classes such as fully-electric trucks which are still at an extremely nascent stage.
AI and ML are also crucial for making smart recommendations regarding the charging infrastructure for EVs. These intelligent systems can take into account the preset destination and combine that with the nearest charging destination with respect to the runtime battery needs of the vehicle. AI and ML based models can expertly gauge the chemical health of the battery and make appropriate recommendations regarding slow and fast charging.
The commonly-held perception is that EVs are less prone to failure than ICE vehicles due to a limited number of moving components. However, a closer look reveals that though the number of moving parts has come down, the susceptibility to breakdowns resulting from failure of minutest parts is much higher. This predicament can prove to be a major hurdle on the road to EV adoption. Therefore, leading OEMs are investing heavily on designing machine learning systems which can make accurate predictions regarding the health of EV drivetrain components by simulating environmental conditions to avert any critical damage like battery heating and motor-lubrication failure. By accurately gauging any looming wear and tear or damage in internal parts, these hyper-intuitive predictive platforms can alert both the owner of the vehicle as well as the OEM to circumvent breakdowns.
With AI and ML moving from the Cloud and onto mainstream applications, it can be safely asserted that these new-age tech paradigms are on the edge. With computational ability advancing exponentially, present-day vehicles come decked with advanced processors that can seamlessly execute sophisticated AI and ML programs using on-premise, edge computation. Today, AI and ML can monitor even the minutest details regarding driver behavior such as drooping eyelids and level of alertness, safeguarding against fatal accidents. These next-gen microprocessors which are embedded deep in the vehicle also enable adaptive cruise control and autonomous driving ability that can significantly decrease driver exhaustion.
Currently, scientists are also dabbling with low-fidelity microcontrollers for running TinyML. These AI and ML micro controllers can greatly optimise the cost of enabling AI for applications such as collision warning, lane departure alerts and reading traffic signs. At the center of this revolutionary automotive trend, is the MCUNetV2, a first of its kind deep learning framework which consists of Convolutional Neural Networks, computed in a patch-wise manner. This latest technology has the potential to redefine the face of the automotive industry.
Safety continues to be the ultimate focus for OEMs, worldwide. Implementing AI and ML can also help in assessing a wide pool of erroneous driving habits which can increase the risk of accidents and reduce energy efficiency, while making recommendations to avert the same. With the rise of ADAS or advanced driver assistance systems, most vehicles today come with active cruise control abilities which use both camera and radar technology to identify vehicles up ahead and isolate any surrounding obstacles by automatically slowing down the vehicle. The emergence of autonomous parking enables drivers to park vehicles seamlessly in tight spots by simply putting the vehicle parallel to the empty slot while the AI takes care of the rest.
AI enabled cameras focus directly onto the face and determine whether the driver is intoxicated by employing various metrics such as breathing patterns and blink rate which changes drastically whenever the driver is drunk or lethargic. ML based instrumentation can also detect the level of alcohol related vapor in the driver cabin. Come 2022, these AI based sensors will also be able to distinguish whether the ethanol inside the car is sourced from liquor or everyday items like room freshener and sanitisers.
Both AI and Ml also assert an enormous role in high octane motorsports like Formula One and Formula E for commencing various designing and racing decisions. It is common knowledge that all major formula one outfits like Ferrari and Mclaren leverage AI and ML to train systems for making crucial racing decisions pertaining to cornering settings and design decisions pertaining to the aerodynamic package such as angle of wings, contour of the wings and undercarriage diffusers.
AI and ML models are also increasingly being used to ascertain fuel quality at diverse locations. For instance, the quality of fuel that you would get in a metropolitan will certainly be different from what you will get in a tier-3 town. The AI can evaluate trends pertaining to metrics such as Mean Time Between Failure (MTBF) for failures in the fuel injection system and make recommendations around locations where the quality of fuel may be sub-standard.
The growing pertinence of AI and ML in everyday life is undeniable, as both have the potential to redefine every aspect of it. In the case of the automobile industry, AI and ML are proving invaluable in mitigating a number of challenges, especially with regard to EV adoption and developing planet–friendly automotive systems that not only promise never-before-seen levels of performative efficiency, but also enhance user safety.
Author:
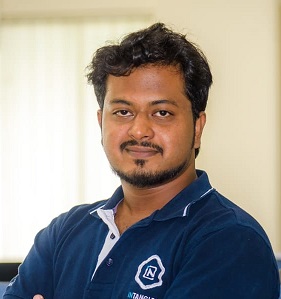
Mudassirkhan Pathan
Lead Data Engineering and Co-Founder
Intangles Lab Pvt. Ltd.
The author is the Lead Data Engineering and Co-founder, Intangles Lab Pvt. Ltd. He is a philomath by heart and a technology aficionado. He is a flag-bearer of perfectionism with comprehensive knowledge of Software Architecture & Problem Solving. Mudassir brings out-of-the-box expertise in Data Engineering. He has done his Bachelors in Computer Engineering from PICT, Pune.
Published in Telematics Wire