How everyone can gain from sharing data
As the world is constantly changing, maps need to be updated continuously as well. Today and in the future, autonomous vehicles will be heavy consumers of up-to-date map data. The question that arises then is how this is supposed to work since traditional ways of mapping have a hard time keeping up with these needs. The more people, organizations, and companies could add value, the better. If that kind of data was available to everyone, maps could be kept updated all the time to ensure safer and smoother rides in the autonomous vehicles.
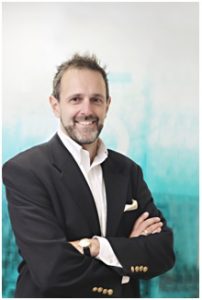
Teaching the car to see
As humans, we take in a lot of data about our surroundings when we’re driving: how other cars, cyclists, and pedestrians are moving, the street signs that we see, as well as other parts of the traffic scene such as toys lying around close to a road. Based on all this and combining it with our past experience, we can anticipate potential hazardous situations (e.g. a child running onto the street) and adapt accordingly.
Autonomous vehicles will need to go through the same chain of taking in a lot of data, processing it, and then making decisions based on that. To get somewhere close to the experience of human drivers, autonomous vehicles initially need a lot of data so that the algorithms that drive them can be properly trained to recognize different objects that may occur in traffic situations such as vehicles, pedestrians, lanes, etc. For this, we need training data from different locations and different scenarios like time of day, weather conditions, and season.
Diversity is key when training algorithms as traffic scenarios could differ quite a lot between continents and countries, e.g. there will be cyclists on the narrow streets in Amsterdam while they would be sparse in other street scenes like Los Angeles. Also, as cities are constantly changing, there is a lot to be won from having diverse data so that you can prepare for not just today but for the future as well.
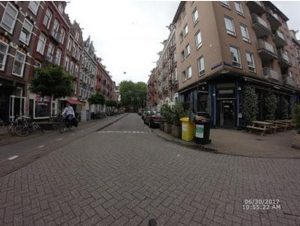
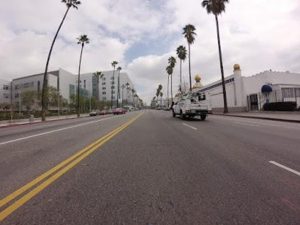
The more data sources you have and the more diverse they are, the better your algorithms will be. But this is also what makes it hard to gather enough images on your own. A collaborative approach could help solve this by creating a diverse dataset that covers all kinds of scenarios.
How to get the best training data
Imagine if you could get access to street-level images from a platform that anyone can contribute to anywhere on the globe, regardless of what type of camera they use (e.g. mobile phones, action cameras, professional surround-view camera rigs as well as embedded automotive cameras). Then you would be able to find the different images needed for algorithm training, based on location, time and date, and what types of different objects are found in the images.
When you have selected the images you want, they need to be manually annotated and labeled by humans and then you have your dataset ready for training vehicle algorithms to recognize different types of objects and understand street scenes. There are different datasets available out there, but the Mapillary Vistas Dataset (http://www.mapillary.com/dataset/vistas) is the only one constructed from a collaborative pool of imagery, and as a result is one of the most comprehensive available for this kind of AI training.
Today, there are many different types of contributors to Mapillary’s platform. Hobby contributors would like to share their work. OpenStreetMap volunteers capture and use the images when editing maps. Cities like Amsterdam and countries like Lithuania share their imagery for public access and the chance for others to develop services and applications upon it. Companies share their existing imagery for disaster recovery purposes like Microsoft sharing images from hurricane-affected areas in Florida and Texas. And so on—different immediate purposes, but sharing means that everyone benefits from more than they contribute just by themselves. And that’s what motivates everyone to collaborate on the same platform, creating a very rich pool of data.
From training to the real world—getting map data
After we have built up an imagery database, enabled everyone to contribute to it, and also created a dataset from the imagery that could be used for training algorithms, what’s next?
Now it is time to extract data from all the images. What kind of data would be of interest when we think about autonomous vehicles? The obvious ones are traffic signs, both to find all of them in a street scene and to understand which one is which. In addition, there is a lot of other information in the images that is of interest, such as guardrails, sidewalks, mailboxes, and fire hydrants. The computer vision algorithms at Mapillary are trained to recognize 97 different object classes, including also moving objects that are not very common such as caravans, or objects that are in the street scene only temporarily such as temporary traffic lights.
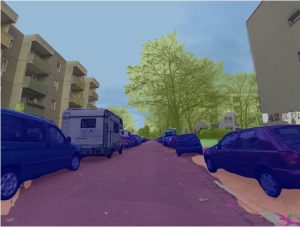
What is the use of all this data extracted from the images?
In addition to the ability to understand the world around them, autonomous vehicles also need maps to help them navigate. The more detailed the map, the better it helps the vehicle to understand its surroundings.
When building an HD (high definition) map for an autonomous vehicle, the starting point is a base map—the underlying framework. But the key differentiators in the future will not be the different base maps but rather the kind of map data (such as map objects derived from geotagged imagery) that takes the map from SD (standard definition) to HD..
This kind of map data has traditionally been collected with help of dedicated fleets of vehicles equipped with sensors and cameras that gather information and map a certain area by driving through an it street by street. There are limits to this kind of approach though, as it doesn’t scale above the number of mapping vehicles. So the data becomes outdated quickly—if it’s available at all.
Scaling it up
Just like with computer vision training data, more is better also when it comes to map data. The fresher and more extensive the HD maps of an area are, the better the navigation experience will be. This is why the best approach to gather data is a collaborative one where anyone with some kind of camera with GPS can contribute. This helps mapping companies expand the areas they can cover in HD and also keep existing coverage updated.
The approach doesn’t compete with what map providers are doing with their own mapping vehicles and maybe even with their own crowd-based solutions, if they should have those as well, but rather complements it. Remember, more data means better maps. It also keeps the costs down as it is not cheap to drive around with a dedicated mapping fleet. Last but not least, the same data can be used for lots of other applications besides making HD maps for autonomous vehicles. So collaboration and data sharing lead to more innovation, faster progress, and lower costs for everyone. It creates a network effect where everyone benefits from contributing to the same pool.
With that vast amount of images, computer vision is the only scalable way to get map data. All images get processed in Mapillary’s cloud, and as a result of combining object recognition with 3D reconstruction, map features are automatically extracted and then included in map update packages that are available to OEMs and map providers. To give an overview of the flow in the Mapillary cloud, see the image below.
Some final words
To summarize what Mapillary does:
- Building a collaborative street-level imagery platform that anyone can contribute to using a wide variety of hardware (cameras and GPS systems) for image/data collection
- Using computer vision technology to extract map data from imagery
- Helping train autonomous vehicles with diverse datasets
- Providing fresh and comprehensive map data that helps autonomous vehicles to navigate
The technology is there, so the final question is whether the automotive world is ready for collaboration on data collection, regardless of suppliers, camera hardware, and base maps used. Hopefully yes, so that we can enable all autonomous vehicles get out there soon, safe for everyone.
Author: Emil Dautovic, VP Automotive, Mapillary
Emil is leading Mapillary’s automotive initiative for providing data and services for the future of cars based on Mapillary’s street-level imagery platform that is powered by collaboration and computer vision.
He has been involved in automotive related business for more than a decade, starting at TAT The Astonishing Tribe building up that business internally from scratch and thereafter joined the BlackBerry subsidiary QNX to drive the business development for the European Market. Emil holds a Master of Science degree in Electronical Engineering from the Faculty of Engineering at Lund University, Sweden.