Data Annotation for Autonomous Vehicle Technology
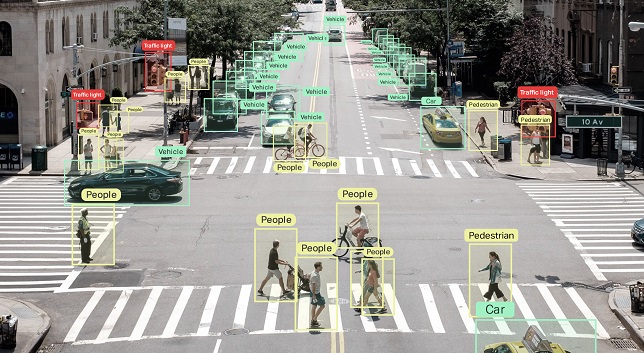
The number of players innovating and developing autonomous vehicle (AV) technology is on the rise. This is a significant indication of the immense potential of AV technology globally. The recent Autonomous Vehicles Readiness Index (AVRI) ranked the US fourth, behind Singapore, the Netherlands and Norway. However, it ranked second in innovation behind only Israel. India is at a nascent stage, but striving with great potential to explore AV technology. This further justifies the number of startups and companies building AV technology across the globe, especially in countries like the US.
AVRI is a score devised by KPMG assessing a country’s current readiness for incorporating AVs onto their roadways. The AVRI rates countries on several different aspects, from innovation to infrastructure.
Many countries are taking note of the rising need for and potential of AV technology, and building initiatives to nurture its growth. For example, the US rolled out a $1 trillion infrastructure bill that makes numerous suggestions for modernizing infrastructure to facilitate widespread adoption of AVs and mobility. Still, manufacturers and innovators need to master the art of crafting models to perform on any road.
AV needs to navigate a highly dynamic environment and face numerous unique challenges referred to as ‘edge case scenarios’. Thus, along with infrastructure, key to the success of AVs, innovators must focus on high-quality data and expertly implemented artificial intelligence (AI) models to predict and assess edge cases.
Heightened focus on AV safety
You might wonder about the need for AV technology. To understand the rationale, it is important to look at vehicle safety compromises and road safety breaches around the world. These have caused 1.35 million deaths due to automobile crashes till 2018, with a person at the wheel, according to the World Health Organization (WHO), making it one of the leading causes of death for people aged 5 to 29.
Significantly reducing this number is one of the biggest challenges we face in the 21st century, with modern transportation at an all-time high. Globally, car makers, software companies and others spent more than $54 billion on AV development in 2019, and market researchers expect that to grow more than tenfold to $556 billion by 2026.
It’s not surprising that the sector has attracted many global leaders in AI, software development and device engineering.
Data annotation and AV safety
When we compare a car driven by a computer to a car driven by a person, we are comparing perspectives. According to the US’ National Highway Traffic Safety Administration, there are more than six million car crashes every year. More than 36,000 Americans die in these crashes, with another 2.5 million ending up in hospital emergency rooms. The global figures are even more staggering.
One could ponder if a switch to AVs would cut these figures drastically. But those involved in various AV initiatives admit that the more revealing characteristic is consumer confidence. How willing are consumers to consider opting for a fully autonomous vehicle, or be ferried around in one?
A 2018 Rand Corporation report, ‘Measuring Automated Vehicle Safety’, studied the conflict between the need for empirical data for AV developers seeking to advance the state of the art and the determination of consumer safety regulatory bodies to resist what they generally regard as avoidable risk. It said: “In the United States – and elsewhere, to some degree – the emergence of AVs has been associated at least implicitly with the view that some exposure to risk and uncertainty about this risk must be accepted in the short and medium terms to see the long-term benefit of AVs.”
Importance of mapping
AVs need to be safer than human-driven cars – that is the ultimate goal. It can only be achieved if all technologies deployed are complimentary – mapping, sensors, AI and predictive intelligence.
Mapping is key. AVs require a mix of directions, roads, traffic conditions, street imagery and other directional characteristics to make good decisions while running the algorithm. And all this is required in real time. We are expecting AV technology to predict and improvise decisions based on what is happening around the car.
To achieve valuable data at such a granular level requires intelligent tools and technology. For example, Tesla counts on video-based systems whereas most AV makers employ LiDAR and video for the data they need. The former provides location accuracy, and the latter adds depth perception.
LiDAR is accurate within centimeters and can create 3D maps for vehicles with a sensor range of around 200 metres. LiDAR does not demand ambient light and is less sensitive to rain or fog through its use of near infrared signals. Apple, Ford, Volkswagen, Microsoft, Hyundai and others are investing heavily in LiDAR, transforming the research into an arms race as most explore it.
As a global leader in data annotation for autonomous vehicles and having enriched more than 150 million data points, we affirm that data is critical for AI models to work precisely. Since autonomous vehicle development is largely a visual interpretation task, all the training data is some form of video – from still images to full-motion video. Cameras and other sensors installed in an AV are bombarded with constantly shifting streams of information. Some of it is static – buildings, fields, lampposts and the like as the car travels down a street or highway – while the rest represent seemingly random events that can require immediate intervention by the car’s AV computer – a pedestrian darting out between parked cars, a bicyclist cutting in front of or around the car or another car veering into the same lane.
In each instance, the AV algorithm controlling the car must make split-second decisions about the nature of the object and the danger it represents to the vehicle – or vice versa.
Types of data annotation
Simply put, data annotation is the process of tagging or classifying objects captured in a frame by an AV. This information is further curated to feed deep learning models and labelled or tagged manually or using AI models or a combination of both. This process is required to help AVs to learn to detect patterns from data and classify accurately to make the right decision. It is also important to deploy the right type of annotation to acquire optimal data. Here are the types of data annotation for AVs:
- Bounding Box Annotation: Marking rectangular boxes to identify targeted objects
- Semantic Segmentation: Annotating images after segmenting into component parts
- 3D Cuboid Annotation: Using 3D cuboids to illustrate desired objects by judging camera perspective to obtain spatial-visual models
- Keypoint/Landmark Annotation: Determining shape changes by multiple consecutive points
- Polygon Annotation: Annotating an object’s exact edges, regardless of shape
- Polyline Annotation: Marking lines to define pedestrian crosswalks, single lanes, double lanes, etc, for road recognition
- 3D Point Cloud Annotation: Annotating 3D point clouds to support LiDAR and radar
- Object Tracking: Locating and tracking objects across a series of images or point clouds in sequential frames
- Instance Segmentation: Identifying each object instance of each pixel for every known object within an image
- Panoptic Segmentation: Coupling instance and semantic segmentation
- Multi-Sensor Fusion: Combining LiDAR, Infrared and images from multiple angles captured from different sensors
Edge cases
The progress of AV technology has been substantial in the past decade. However, the technology still faces a massive barrier that is preventing mass adoption. This barrier is known as an ‘edge scenario’, a unique situation or unusual occurrence in which an AV is unable to appropriately address or identify an unusual obstacle, circumstance or incident on the road, which can result in an accident or fatality.
As compared to a fully focused human driver, AVs lack the intelligence to register and react to random occurrences and developments. To address edge cases, AVs require special design considerations to handle these developments securely.
As data is largely generated manually for the training of AVs, it is challenging to train vehicles to perform for unlikely happenings that may take place on the road. It is difficult for a system to perceive the environment like a human.
Environmental perception is a contextual understanding of the ecosystem or the situation, such as locating obstacles, recognition of road signs/markings, and sorting data by their semantic meaning. A trained model can predict many edge cases, and edge case training can make the autonomous system more robust under novel operational conditions.
For instance, a road sign with an image of a deer can be confusing for an AV. It may treat the sign as an object and stop abruptly. A person with a trolley or a pram on the road might not be identified by the car. It is crucial to factor a balance between analysis and field experience because an edge case, like cattle on the road, could be a single point of failure if not trained.
Each country, city, and landscape present a unique set of edge cases and training for these challenges – a constant challenge itself. Situation is an essential element when defining the nature of any roadside development or sign. For example, every country/city’s police department has its own distinct appearances and every city/country has unique road laws. While cattle on the road may be commonplace in certain parts of the world, they might not be in others.
The most significant safety benefit of an AV is that it is not a human. It is designed to follow all traffic laws and to be indestructible by minor things like text messages or flashing phone screens. AVs can also detect what humans cannot, especially edge cases, and respond more rapidly to prevent a collision, at least in theory. Accurate labeling of edge case data helps push this from theory to reality.
Edge cases are a massive deterrent to the mass adoption, safety and efficiency of Avs. Predicting and addressing them is an essential element of success for AVs. With the right workflow, talent, and understanding of a region’s given edge cases, they can be overcome effectively.
The future
The push for AVs has led to massive innovations and driverless cars are already out on some roads, revolutionizing travel. To continue this rate of development, innovators are continually going to require access to high-quality, affordable data. This is an enormous opportunity for data annotation experts like us to collaborate with people, process and technology to deliver the best datasets. For AVs to become a common reality, data annotation providers and developers must innovate to resolve edge cases and build data-driven systems which are foolproof and perceptive.
Author:
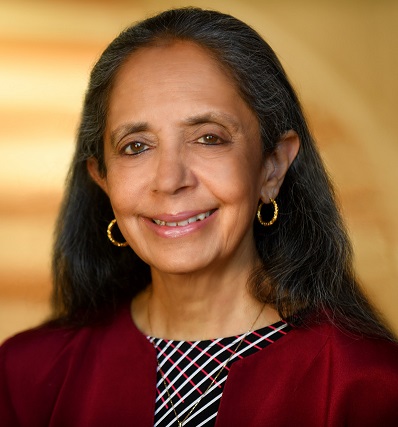
Radha Basu
Founder and CEO
iMerit
Radha Basu is the Founder and CEO of iMerit, a global AI data solutions company delivering high-quality data that powers machine learning and artificial intelligence applications for Fortune 500 companies. She is a leading tech entrepreneur, and a pioneer in the Indian software business. Under her leadership, iMerit has employed hundreds of skilled and marginalized women and youth in digital and data services worldwide.
Published in Telematics Wire