Changing Paradigm of Simulation in Automotive Industry
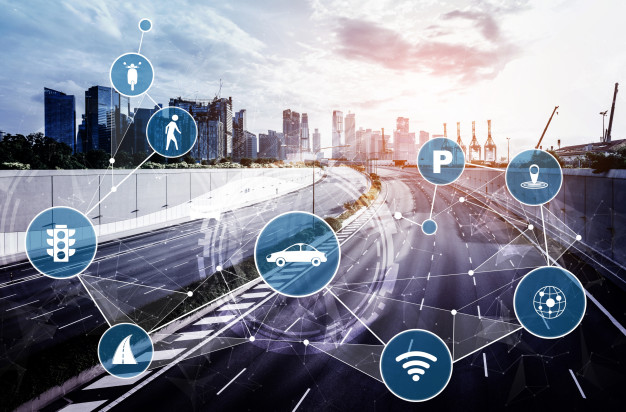
In 2017, Japan released its ‘Society 5.0’ blueprint, which envisions a ‘super smart society’ in which digital tools solve challenges such as the nation’s aging workforce and global pollution. We are now in a new era, one in which innovation driven by enabling technologies such as IoT, AI, and robotics are bringing significant changes to the economy and society. Today, movements toward incorporating new elements into existing technologies and knowledge, thereby designing, and creating previously unknown business services, are gaining visibility.
Digitization, increasing automation, and new business models have revolutionized other industries, and automotive is no exception. These forces are giving rise to four disruptive technology-driven trends in the automotive sector: diverse mobility, autonomous driving, electrification, and connectivity or collectively known as CASE (Connected, Autonomous, Shared and Electric). The number of vehicles with CASE mobility characteristics will multiply over the next decade, completely changing the automotive landscape. The automotive companies are striving for speed to market and new technologies are bringing value through customized solutions catering to individual choice. To address this challenge, they must begin thinking beyond their traditional benefit offerings and overcome the deterrents standing within the way of speedy adoption of new technology by digital transformation to stay afloat in the market. The onset of COVID-19 has accelerated this process due to multiple factors with people working remotely, and this gives each of us a chance to re-imagine and think how we could do things differently. They are giving jitters to automotive executives who are worried about the rising complexity of vehicle engineering. The chances of an engineer failing to uncover and address potential catastrophes are directly proportionate to a vehicle’s design complexity. One of the key tools to address this challenge is “Simulation”.
Simulation allows automakers to virtually test and analyze an entire vehicle and its parts even before physical prototypes are made. It can help optimize a vehicle for safety, fuel economy and passenger comfort. The automotive industry was an initial adopter of simulation and has been leveraging this technology for the design and development of vehicles for several decades. In India, by skipping BSV and jumping directly to BSVI, the challenge of reduced time to adherence and technology development to fit the market made it imperative to develop simulation techniques to reduce the time and cost for physical testing. Moving simulation upfront in the product development cycle, finalizing combustion recipe by predicting performance and in-cylinder emission parameters, finalizing Coolant Hardware architecture, and achieving durability mileage and life – all these have been possible by applying simulation and developing simulation methodologies to address complex real-world scenarios and regulation requirement.
Though we have made significant progress in individual physics areas and models utilized for single discipline optimization, CASE vehicles pose multi-dimensional, multi-variant, multi-domain challenges. To address this, we need to break silos, create cross-functional integration and develop model-based engineering approach that uses models as an integral part of the engineering processes that includes the requirements, analysis, design, implementation, verification and validation of a system throughout its lifecycle.
Model based System Engineering (MBSE)
Model-Based Systems Engineering (MBSE) is the practice of developing a set of related system models that help define, design, and document a system under development. These models provide an efficient way to explore, update, and communicate system aspects to stakeholders, while significantly reducing or eliminating dependence on traditional documents. MBSE is the application of modeling systems as a cost-effective way to explore and document system characteristics. By testing and validating system characteristics early, models facilitate timely learning of properties and behaviors, enabling fast feedback on requirements and design decisions. MBSE helps the system engineer in assessing the relevance of the system architecture and the compliance with the desired system properties. The same results can be expected from its application to Simulation Systems.
Logistical complexity and software costs are higher. Higher fidelity designs earlier in the acquisition cycle saves program cost and schedule, design problems and conflicts are caught early and often reduces errors and delays at handoffs between disciplines, it helps capture and re-use of multidisciplinary engineering knowledge and reduces design cycle time by 2x to 3x. It allows models at different levels of fidelity to be integrated, ensures design consistency and captures design history, prevents over-design through high fidelity view of integrated product performance, improves decision making by enabling spatial comparisons between all relevant engineering data and creates ownership for overall product success among team members
Digital Twin
Today, detailed physics simulation is used to optimize structural, fluid flow, electromagnetic, thermal, and other physical properties. However, on ground, only a few are operating under the conditions envisioned when they were designed. Until recently, the people responsible for keeping these assets running at maximum efficiency had no way to understand the effects of operational and environmental changes on asset performance. Is the machine headed for a breakdown that could cost tens of thousands of dollars per hour in lost production? Is it being run at sub-optimal conditions that will reduce its life over the long run? Are there opportunities to improve its performance by making changes to its operating conditions or upgrading its capabilities?
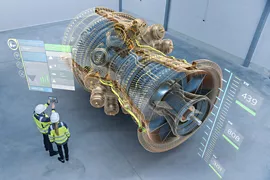
Technologies for engineering simulation and the Internet of Things have revolutionized asset management with a concept called the digital twin. Companies have been using sensors to collect data for many years especially for the high asset machine. However, this data is not always collected in real time, and the vast amount of data has made it difficult to extract actionable insights. The Internet of Things (IoT) makes it possible for the first time to use sensors to capture data from these assets to understand and optimize their performance instantaneously. By combining this operational data with other information on how the machine works — including maintenance records, PLM information and simulation results — together with analytics and machine learning to form an ecosystem, a fully featured model called a digital twin can be built. Using a digital twin, it is possible to diagnose complicated problems that involve interactions of multiple subsystem and factors.
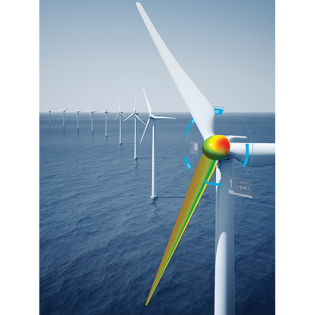
Simulation is critical to a digital twin as it supplies answers to questions like “What if we change this?” and “Why did that happen?” and “How do we improve the design?” Merging physics-based understanding with analytics delivers the insights that unlock the true value of the digital twin. Using these insights, engineers can understand the operational failure modes of the product, prevent unplanned downtime, improve product performance, and seed the next product generation. Digital twin promises to extend the value delivered by simulation beyond product development to the entire life cycle of a product enabling it to be studied under its actual operating conditions in its unique working environment. By creating a replica of the actual product system in a digital environment, engineers can anticipate and address potential performance and maintenance issues before they occur. The real-time, real-world insights collected via these digital twins can also accelerate future design iterations, leading to continual product improvements. Simulation-based digital twins complement analytics as you can generate baseline and failure data with simulation and predictive models provide higher accuracy and improved ROI by re-using existing simulation models. In addition, the operator can perform what-if analysis to simulate different solutions before applying to the assets. To maximize the value of simulation, the smartest engineering teams apply it at every stage of the development cycle.
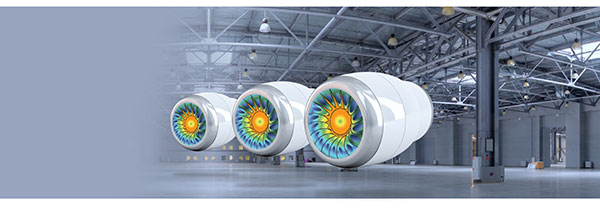
Conclusion:
Engineering simulation has already demonstrated significant financial payback for companies. It has helped cut time and cost from the product development cycle, deliver quality products, and reduce service and maintenance expenses, resulting in higher customer satisfaction. The availability of compute resource power, coupled with technologies like IoT and AI/ML with focus on Model based system engineering are all leading to a new era of digital transformation. The impact of this transformation is not just a financial gain, but a reputational advantage with a system within the company where greener practices are no longer optional, and achieving carbon neutrality goals is an eventuality.
Author:
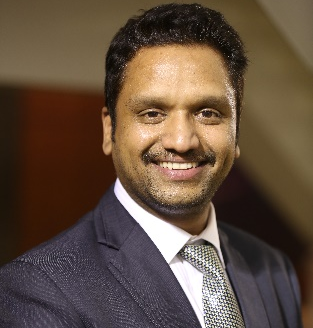
Sandeep Shetty
Technical Manager- APAC
Ansys
Sandeep Shetty is the Technical Manager for APAC Enterprise accounts at ANSYS. In his current role, he focuses on Technical engagement with global Ansys Enterprise set of accounts. He has over 15 years of experience in automotive domain specially focusing on simulation driven product development. He works with customers to help them accelerate product development, process integration and continuous improvement.
Published in Telematics Wire