Artificial Intelligence & Machine Learning applications for 5G and beyond
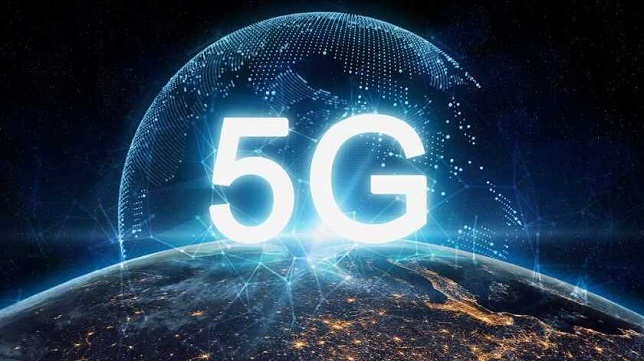
Introduction
Increased digitalization supported by a well-established wireless communication system plays a critical role in societal growth to offer multiple applications and services including communications, connected vehicles, healthcare, travel & tourism, entertainment, and last-mile connectivity. The fifth generation (5G) of wireless networks is being deployed across different countries, including India. Artificial Intelligence (AI) synergistically enhances 5G networks by delivering new capabilities and offering new business opportunities. The proliferation of 5G-connected sensors, devices, and vehicles in an Internet of Things (IoT) or Internet of Vehicles (IoV) environment, accompanied by AI advancements, can help improve 5G system performance and efficiency, along with distributed intelligence across the connected entities. AI-enabled 5G, with inherent advantages such as software-defined networks (SDN), network function virtualization (NFV), ultra-low latency, high security, and ultra-high reliability, not only enhances the perception, reasoning, and decision-making ability of the devices but also offers a new paradigm for problem-solving approach. The new business opportunities and use cases force the network operators to increasingly use AI in their networks to deeply and accurately learn the evolving system needs and consumer behaviour.
AI enhances the inherent strength of 5G networks by providing autonomous capabilities which allow the networks to evolve into self-organizing networks (SONs) that perform most functions without human intervention, adapt themselves to changes in the network conditions, and repair faults when they occur (Ref [1]). The accuracy of AI algorithms and models depends on a large amount of data for training and implementation which is well enabled by the 5G support for massive device connectivity and IoT networks via massive machine-type communications (mMTC). As AI algorithms demand high levels of computational capabilities supported by increased memory and better processors, 5G networks provide a suitable environment through the inclusion of caching and edge computing. With software-defined network (SDN) capabilities along with its associated technologies such as Network function virtualization (NFV), network slicing, etc., 5G networks would also facilitate the use of AI techniques for intelligent and dynamic network management and orchestration. AI also plays an important and expanding role in the evolution of 5G towards 6G.
Keywords: Artificial Intelligence, Machine Learning, Next Generation Mobile Networks, 5G, 6G, Edge Cloud, Deep Learning, Mobile Computing
AI & ML Applications across different industries
Over the past decade, many industries have benefited from the applications of Artificial Intelligence (AI) and Machine Learning (ML). In recent years, as the COVID-19 pandemic has disrupted usual life and work patterns and caused severe economic losses across different sectors, increased digitalization aided by 5G and AI together contributed to speeding up the evolution of a fully connected and intelligent world. Figure 1 shows the role that 5G technologies play(ed) in various sectors to enable them to handle the challenges caused by COVID-19 pandemic by supporting various operations or technologies in each sector (Ref [1]). As Figure 1 (Ref [1]) indicates, 5G technologies such as enhanced mobile broadband (eMBB), ultra-reliable low latency communications (URLLC), and massive machine-type communications (mMTC) enabled different sectors such as healthcare, education, travel & tourism, manufacturing, and agriculture to recover from the pandemic.
In ref [2], a detailed study is presented on how AI has benefited 13 global industries. The biggest impact of AI across different industries is shown in Figure 2 (Ref [2]) while the biggest revenue gains due to AI implementation are depicted in Figure 3 (Ref [2]). It is evident from figure 2 that the Information technology (IT) function is the most impacted across different industries ranging from banking and financial services to health care and life sciences to travel, transportation, and hospitality sectors. Among manufacturing-oriented industries, AI’s impact is most evident in automotive and consumer packaged goods (CPG). In industries with significant customer touch points through sales and service such as CPG, retail, and utilities, the biggest impact of AI is observed.
Figure 3 clearly indicates that Telecommunications, Hi-tech, Retail, Banking & Financial Services, and Energy are the top 5 industries with the biggest revenue gains due to AI implementation (Ref [2]). The numbers from Figure 3 also indicate that AI implementation resulted in an average revenue increase of 17% with an average cost reduction of 12% though the numbers vary across the industries.
Next-Generation Mobile Networks
Next-generation wireless networks are expected to support a multitude of services across a range of heterogeneous devices with different Quality of Service (QoS) demands and flexible allocation of network resources in response to network dynamics. The use cases for next-generation networks range from rapid transfer of messages among connected & autonomous vehicles (CAVs) with near-zero latency to high-quality Augmented Reality (AR)/Virtual Reality (VR) entertainment applications.
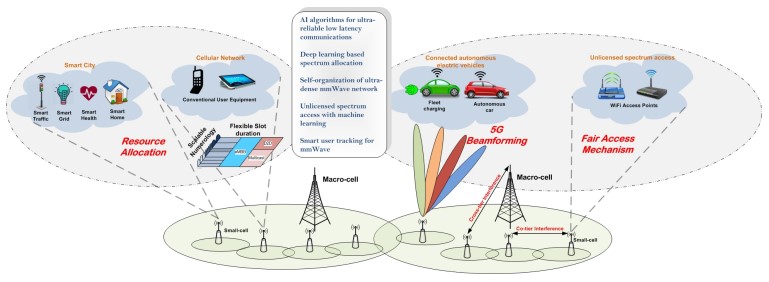
AI-enabled next-generation wireless networks, shown in Figure 4 (Ref [3]), cater to diverse use cases such as dynamic resource allocation for smart city applications, CAVs, deep learning (DL)-based spectrum allocation for cellular networks to handle heterogeneous traffic coming from mobile, vehicular, smart grid and tactile domains, and self-organizing networks (SONs) using ultra-dense mmWave spectrum. To meet the stringent demands for resource efficiency, reliability, and robustness for 5G and beyond networks, AI/ML techniques are deployed to intelligently manage the networks and the resources (Ref [4]). The diverse use cases for 5G and the associated lessons learned in implementation pave the way for advanced technologies toward 6G network design and standards development.
AI use cases in 5G & beyond
Recent reports and continuing research indicate that 5G networks provide unifying connectivity with virtually everything around us through connections/communications with billions of IoT devices. Artificial Intelligence is a learning platform that can make virtually everything around us intelligent.
Different ML algorithms such as Supervised Learning, Unsupervised Learning, and Reinforcement Learning apply different models and support specific applications in 5G. Below is an excerpt of different learning classes and corresponding applications in 5G (Ref [5]).
Learning Classes | Learning Models | Applications in 5G |
Supervised Learning | ML and statistical logistic regression techniques Support Vector Machines (SVMs) | Dynamic frequency and bandwidth allocation in self-organized LTE dense small cell deployments Path loss prediction model for urban environments |
Unsupervised Learning | K-means clustering, Gaussian Mixture Model (GMM), and Expectation Maximization (EM) Hierarchical Clustering | Cooperative spectrum sensing and Relay node selection in vehicular networks Anomaly/Fault/Intrusion detection in mobile wireless networks |
Reinforcement Learning | Reinforcement Learning algorithm based on long short-term memory (RL-LSTM) cells Reinforcement Learning with Network assisted feedback | Proactive resource allocation in LTE-U Networks is formulated as a non-cooperative game, which enables SBSs to learn which unlicensed channel, given the long-term WLAN activity in the channels and LTE-U traffic loads Heterogeneous Radio Access Technologies (RATs) selection |
Qualcomm showcases demos on how AI can benefit the performance and efficiency of 5G operating in sub-7 GHz and mmWave across different use cases (Ref [6]). The use cases in 5G and beyond with AI deployment include the following and are shown schematically in Figure 5 (Ref [6]).
- Massive MIMO channel state feedback – provides higher throughput and improves system capacity
- Mobile mmWave beam prediction – helps improve capacity and extend battery life
- Positioning accuracy – improved through a combination of 5G, GNSS, multiple sensors, and varying path-profiles
- Network planning – diverse node types used for the efficient improvement of mmWave coverage
- AI-assisted RF sensing for indoor positioning – currently Wi-Fi is used with future applications planned to use 5G
In Ref [6], it has been well captured on how AI and 5G are both working together to accelerate innovations. This is shown schematically in Figure 6, wherein
- Advances in AI result in the betterment of 5G with the following benefits
- An enhanced level of performance
- Highly efficient resource utilization
- Improved battery life through optimal energy consumption
- Data privacy protection and personalized security
- Kaizen (Continuous improvement)
- Proliferation of 5G makes AI applications better with the below benefits
- Positively responsive user experiences and services
- Continued lifelong learning
- Improved flexibility for distributed functionality across devices
- Cloud-based enhancement of intelligence at the edge devices
- Distributed learning-assisted intelligence scale up
- Large data availability for improved AI models
On-device AI improves the 5G end-to-end system. Figure 7 shows the evolution of 5G, AI, and cloud from cloud-centric AI in the past to partially distributed AI in the current scenario to fully distributed AI in the future. Among different ML algorithms, Federated Learning (FL) is found to bring on-device learning to a new level. The evolution of Connected Intelligent Edge through the mutually beneficial cycle shown in Figure 6 is presented in Figure 7 (Ref [6]). Recent studies confirmed that processing data at the edge devices provide improved key performance indicators (KPIs) such as ultra-low latency, enhanced privacy, and improved cybersecurity. To achieve higher levels of efficiency and scale-up of AI-enabled intelligence, more data processing needs to be taken up at the edge on devices such as smartphones, cars, laptops, and smart wearables (watches, glasses, and biometric sensors). Connected Intelligent Edge brings new and enhanced devices by combining edge cloud AI with on-device AI to provide multiple services such as a) contextual awareness, b) fingerprint-based security, c) voice activation, d) face detection, e) gesture tracking, and f) computational photography, to name a few. These services facilitate multiple intelligent edge opportunities such as a) local network analytics, b) low latency interactive content, c) industrial automation and control, d) on-demand computing, Augmented Reality/Virtual Reality, etc.
Towards 6G
Machine Learning (ML) plays an important and expanding role in the evolution of 5G towards 6G. AI-enabled 6G networks will a) provide high throughput, b) support new high-demand applications, and c) improve the usage of radio frequency bands, to name a few. 6G wireless connections for data exchange at the various spectrum of frequencies using different cutting-edge technologies. Researchers have been building upon 5G systems to conceptualize 6G, where new 6G wireless systems will have ML as their main component for the physical layer, transceiver design, network, and application layers (Ref [7]). Multiple studies are being carried out on how ML techniques can be
- beneficial in wireless channel estimation and resource allocation
- used to devise better network-layer protocols for next-generation wireless systems
- embedded in various convention layers of a wireless system
A conceptual representation of an ML-based 6G wireless network is shown in Figure 8 (Ref [7]) which depicts how ML and AI will be embedded in various conventional layers of a wireless system and play a key role in system performance. The conceptual Open Systems Interconnection (OSI) seven-layer model can be categorized into three main categories:
- Physical layer (sensing layer, network layer)
- Middle layer (data link, network, transport, and session layers)
- Application layer
As shown in Figure 8, across different layers and at different network levels, ML algorithms are to be trained with some given data sets for deployment in base stations (BSs), mobile devices, core, and management layers with network assistance. Owing to the need for different sources of data for different network functions in the management domain, a data-driven and ML-native network will be evolved using these new techniques.
Among the ML algorithms, Deep Learning (DL) is found to provide potential solutions to some of the challenges faced in 6G evolution because of its strong applications in learning and building models from real-life situations. Researchers are continuing to explore what additional roles DL can play in wireless networks based on the earlier successful application in the classification problems, quick decision-making on access points to connect to in 6G, optimal resource controller utilization, etc.
A representative evolution of 6G and the critical role of AI/ML techniques in the next-generation wireless networks is shown in Figure 9 (Ref [7]). In this schematic, efforts are made to develop a baseline knowledge of ML techniques that can be applied at the infrastructure level and user/application level for improved wireless system design such as in channel modelling, channel estimation, and transceiver design. The idea of this concept is to design and build self-organizing networks (SONs) for efficient and effective resource utilization and dynamic scaling as required.
Conclusion
Multiple industries and sectors have been adversely impacted by COVID-19 pandemic. The critical role that 5G technologies play(ed) in various sectors such as healthcare, education, travel & tourism, manufacturing, and agriculture to recover from the pandemic is presented. AI/ML applications across different industries are presented with IT being the most impacted/biggest beneficiary in terms of AI/ML techniques and with Telecommunications, Hi-Tech, and Retails industries being the biggest revenue gainers from AI/ML implementations. Next, AI-enabled next-generation wireless networks were briefly presented followed by specific use cases of AI/ML applications wherein supervised, unsupervised, and reinforcement learning algorithms could be applied. The evolution of cloud computing from cloud-centric AI in the past to the present partially distributed AI to the fully distributed AI in the future was presented next. The mutually synchronous 5G networks and AI/ML techniques with each benefitting the other were then presented followed by a conceptual representation of an AI-enabled 6G network with specific areas of impact by AI at the infrastructure level and user/application level.
Future Work
Next-generation wireless networks (6G) will increasingly focus on “intelligence” though dealing with a higher level of complexity than all the preceding generations of wireless networks. This will necessitate extensive applications of Deep Learning-based algorithms and intelligent mechanisms to orchestrate the available resources, services, and users to evolve into self-organizing networks (SONs). Thus, future networks are expected to employ ML algorithms to learn from their environment, adapt to the changes in an automated fashion, and achieve optimal performance.
References
1. Abubakar, A.I. et. al, “The role of artificial intelligence-driven 5G networks in COVID-19 outbreak: opportunities, challenges, and future outlook,” Frontiers in Communications and Networks, 11 November 2020, https://doi:10.3389/frcmn.2020/575065
2. Ramaswamy, S. “Getting Smarter by the Sector: How 13 Global Industries Use Artificial Intelligence,” TCS Global Trend Study: Part II, 2016, https://www.tcs.com/content/dam/tcs/pdf/Industries/global-trend-studies/ai/TCS-GTS-how-13-global-industries-use-artificial-intelligence.pdf
3. Elsayed M. and Erol-Kantarci M., “AI-enabled future wireless networks: challenges, opportunities, and open issues,” IEEE Vehicular Technology Magazine, vol. 14, no. 3, pp. 70-77, Sept 2019, https://doi:10.1109/MVT.2019.2919236.
4. Kelly P., “AI tackles the challenges of managing the 5G mobile network,” Forbes, March 26, 2021, https://www.forbes.com/sites/forbesbusinesscouncil/2021/03/26/ai-tackles-the-challenges-of-managing-the-5g-mobile-network/?sh=5910a74970e8
5. Haidine, A. et. al, “Artificial Intelligence and Machine Learning in 5G and beyond: A Survey and Perspectives,” in A. Haidine (ed.), Moving Broadband Mobile Communications Forward – Intelligent Technologies for 5G and Beyond, IntechOpen London, 2021, https://doi: 10.5772/intechopen.98517
6. Ji, T, “What’s the role of artificial intelligence in the future of 5G and beyond?”, 21 September 2021, https://www.qualcomm.com/news/onq/2021/09/whats-role-artificial-intelligence-future-5g-and-beyond
7. Kaur, J. et. al, “Machine Learning techniques for 5G and beyond,” IEEE Access, 2021, https://doi:10.1109/ACCESS.2021.3051557
Author:
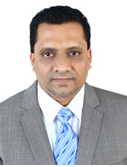
Dr. Arunkumar M. Sampath
Principal Consultant
Tata Consultancy Services
Dr. Arunkumar M. Sampath works as a Principal Consultant in Tata Consultancy Services (TCS) in Chennai. His interests include Hybrid and Electric Vehicles, Connected and Autonomous Vehicles, 5G/6G, Cybersecurity, Functional Safety, Advanced Air Mobility (AAM), AI, ML, Data Analytics, and Data Monetization Strategies.
Published in Telematics Wire