Artificial Intelligence in the Autonomous Vehicle
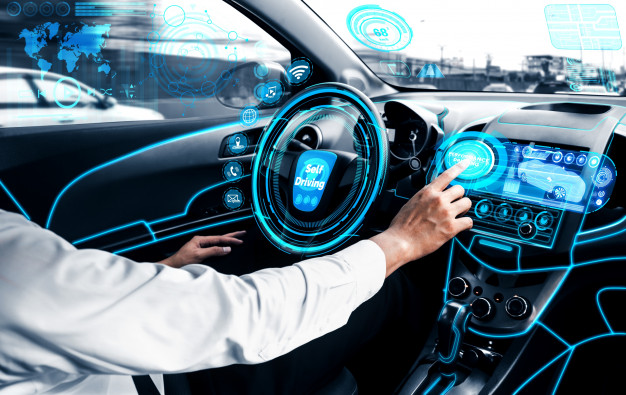
Artificial Intelligence (A) is the hottest technology promising disruptions in every possible industry today. AI is powered by data. Hence, it is not a surprise that AI has begun disrupting the Automotive Industry and the much awaited promise of Autonomous Vehicles because the automotive has begun its journey to become software on wheels and is powered by data from the connected, autonomous vehicle. The data from the car powers the Artificial Intelligence in the vehicle. The mobility of the data in and out of the vehicle determines the type of Artificial Intelligence to be built. The choice of where the AI intelligence resides powers a variety of applications and takes care of the privacy and security questions that arise with data and AI. So to understand AI in the Autonomous Vehicle, it is important to track the journey of the automotive as it navigates through levels of autonomy aided by a wide range of Artificial Intelligence applications and how these applications are bringing the entire technology stack from the world of computers onto the automotive.
Connectivity Leads the Vehicle Towards Level 5 Full Autonomy
The connected, autonomous, shared and electric (CASE) model (see image1 1 below) is the most commonly accepted industry model for the path to full mobility. It was originally proposed by Mercedes-Benz. According to the CASE model the automotive becomes connected and electric to be offered to consumers using shared mobility, leading to full autonomy in the long run.
CASE model for the path to full mobility
The CASE model has prompted Automotive OEMs to continue their journey in digitization of the vehicle by making all models of new vehicles with built-in connectivity with a built-in SIM with carrier connectivity with 4G or 5G where available.
SAE International published “SAE J3016” in 2015 and revised it in 2018 to describe 5 levels of vehicle automation from no automation to full automation. This has become the de facto industry standard following the adoption by the US Department of Transportation. According to SAE Levels, Level 5 is full autonomy where the vehicle can drive autonomously without human support in all road conditions.
SAE J3016 5 levels of Autonomy
Level 5 is the holy grail of full autonomous driving where the vehicle can drive autonomously without the need for a human to take control and it will work in all road conditions. Though every autonomous vehicle company is working to get to level 5, it is a technical challenge that is hard to solve because the Artificial Intelligence (AI) that powers the autonomous feature is a narrow AI that requires training data for specific road conditions to train for all possible humans, other vehicles and other things that would cross the path of a vehicle. This technology area is called perception space and it works by using computer vision for object detection and combines data from several sensors and cameras around the vehicle to get a picture of the road. Level 5 is technically not feasible today because of the need to train the autonomous vehicle for every road in every city in every weather and road conditions while planning for every possible object that could potentially cross the vehicle. AV companies are continuing to build Level 5 while testing new business models with Level 4 and sometimes with Level 3. Level 3 is where a human driver is required to be on standby to take control when needed. Level 3 and 4 are deployed in geofenced geographical areas where the autonomous vehicles are trained. Three business models that are being tested this way include robotaxis for consumer commute, freight delivery in autonomous trucks and middle-mile delivery of goods using small size autonomous trucks or delivery bots. Every one of these applications is powered by AI. Every one of them cannot be built without the support of the technology stack shifting to the vehicle.
The Autonomous Vehicle Technology Stack
The Autonomous Vehicle Technology Stack consists of two main categories of technologies. First is the new AV technology which is powered by sensors and computer vision to facilitate the actual driving. More important is that the entire technology stack from the desktop and mobile is transitioning to move to the vehicle as the car becomes autonomous to become a drive by wire powertrain.
- Car Cognition Technology:
This includes four key modules:
- Sensor Fusion: Cameras, GPS, Radar and LIDAR in the car bring data that are combined to inform the location of the car and what the car sees on the road at any given point in time.
- Perception and maps: Perception is how the car sees the road. This is a map for AVs but more than that. Perception is about understanding the software to take sensor fusion data to make sense of its surroundings. For example, the AV needs to understand if the other vehicle on the front is moving towards it or away from it and understand its vector.
- Localization: Localization is the technology by which the vehicle understands its location relative to the map of its environment. This is true for self-driving cars, or robots and other vehicles powered by autonomy such as AV trucks, autonomous tractors, autonomous forklifts and delivery bots. For the Autonomous Vehicle, or even the AV feature baked in an automotive as an ADAS feature, it is about knowing where the car is relative to the lane markers and other vehicles, shrubs, people and sidewalk. It is not as easy as getting GPS lat-long coordinates as it is used to do motion planning and requires high precision more than the accuracy of a GPS. Localization techniques could measure the distance of the vehicle from every other object it sees in its environment. Another technique is to get a point cloud map of the environment and compare it to what the car sees as objects in real-time. Localization and perception are more about the processing power of this computation in real-time instead of which is a better technology approach.
- Drive by Wire Controls: Path Planning and actuating control to the drive by wire brakes and accelerator is what allows the autonomous vehicle to move, stop or navigate the road to perform the actual driving.
II. The Software-on-Wheels Technology Stack:
These are the technology components that make the car into a software-on-wheels. We can look at them in the context of how they help the Autonomous Vehicle to support a variety of data science applications, connect to smart city infrastructure intra-car or create new design experiences inside the car
- V2X: The data in the car can be from the car sensor or from cameras watching the road to help the car make sense of its surroundings to drive with ADS features or to drive autonomously. This data is transferred to the cloud such as the OEM’s private cloud or smart city infrastructure and in that case it is called V2I or Vehicle to Infrastructure. One example of this is when four different car makers Ford, BMW, Mercedes-Benz and Volvo, partnered to share road safety data using a common cloud. Data can be shared between vehicles to communicate with each other directly and in that case it is called V2V or Vehicle to Vehicle.
- Digital Twins: A Digital Twin is a digital replica of a physical asset that comes from the world of connected devices and IoT. This is essentially sensor data from any “thing” that is collected in the cloud and used to run simulations to manage the physical thing. Now it is used in cars with car parts being tracked remotely to check for health of parts. This is known as the digital twin in the car. There is a movement to build a digital twin of the inside of the car environment to manage the in-car experience and to make a third digital twin of the human themselves in the car to track their moods and behaviours. It is important to consider the ethical issues about passenger agency in the car with this.
Dr. Ashwin Sabapathy in the Telematics wire article from April 2021, titled “Data Science Applications for Automotive Data ” shared driver behavior modelling, risk scoring and accident claim support with accelerometer data as potential data science applications using the sensor data from the vehicle. All these applications are possible only from the digital twins from the vehicle. These promises risk mitigation for insurance companies and potential fuel efficiency with route planning and driver training.
CapGemini Research shows that the top application that the Automotive industry is adopting with AI is Digital/Mobility Services. Examples of such services are preventive maintenance for dealers using data from the connected vehicle or in-car advertisement to offer recommendation to passengers based on their behavioral analytics data.
- Federated AI: Federated AI is the technology of building AI models at the edge as in the car, across multiple organizations to develop a combined shared learning model. The goal is to protect the privacy of the data involved and create a shared model across the industry or partners. Porsche had tested out Federated AI models in the automotive. This technology has the potential to speed car cognition and AI in the car for ethical human centered customer experience in the vehicle across industries.
- Edge AI in the Car or EdgeML: Sometimes the data is used to create design experience for the person inside the vehicle using voice, virtual reality or augmented reality. One example of this is when Mercedes-Benz digitized their driver’s manual using an Augmented Reality experience called ‘Ask Mercedes.’ The data can be stored in the vehicle to personalize the user’s experience. In that case it is called edge intelligence. If Machine Learning models run on the edge, like at the ECU, then it is called EgdeML. In this case, the models learn from the device data and do transfer learning to share their AI learning without transferring data from the vehicle to the cloud to be improved from the learning from other vehicles. Today such edge AI is used in car cognition.
- Data Platform: Edge intelligence can be combined with other data feeds such as weather feeds or location specific information or a retailer’s information to offer advertisement or recommendations for users. These create new business opportunities and have the potential to extend the disruption from the car from automotive to several other industries such as Retail, Insurance, Smart City and Healthcare. Complex data integrations and processing require a robust data platform inside the car. Several Car companies are deploying data platforms in the vehicle to support applications for shared mobility and logistics management using a fleet of vehicles.
- Blockchain: Automakers are experimenting with Blockchain in the automotive to track supply chain and secure data. There is no standard with Porsche working with XAIN, Volkswagen with IOTA and Daimler AG with Hyperledger fragmenting the potential to benefit transportation and autonomous vehicles. The best use case is the secure OTA (over-the-update) which will benefit from Blockchain in the Autonomous Vehicle.
Conclusion: The entire technology stack is in the process of moving to the car. Technology companies have begun competing to offer a mobility platform inside the car and some companies are building data platforms inside the car. AI powers car cognition to drive autonomous driving with many potential applications evolving today with connected vehicles with more potential applications evolving as the autonomous vehicle moves from Level 3 and 4 towards full autonomy. ADAS features and connected automotive capabilities are already offering data science and AI applications as the connected car generated more data with mobility options as the automotive meanders on its journey to become software-on-wheels.
Reference and Reading list:
- What is AV localization by David Silver of Cruise https://www.linkedin.com/pulse/how-localization-works-self-driving-cars-david-silver/
- “Data Science Applications for Automotive Data” by Dr. Ashwin Sabapathy in the Telematics wire article, April 2021
- AV Open datasets and careerpivot webinar resources https://businessschoolofai.teachable.com/p/learnav/
- “AIX: Designing Artificial Intelligence” book by Sudha Jamthe and editor Richard Meyers, Feb 2020 (AIX: Designing Artificial Intelligence)
- “2030 The Driverless World: Business Transformation from Autonomous Vehicles”, Sudha Jamthe, Sep 2017 (https://www.amazon.com/gp/product/1973753677/)
- CNBC “Completely driverless cars are being tested in China for the first time” by Abigail Ng, Dec 2020. (https://www.cnbc.com/2020/12/04/fully-driverless-cars-are-being-tested-in-china-for-the-first-time.html)
- “Taxonomy and Definitions for Terms Related to Driving Automation Systems for On-Road Motor Vehicles, Ground Vehicle Standard J3016_201806”, Revised Published June 15, 2018 by SAE International in United States (https://saemobilus.sae.org/content/j3016_201806) adopted by Federal Automated Vehicles Policy by US Department of Transportation (US DOT)
- Forbes, “Are U.S. Roads Built For An Autonomous Vehicle Future?” by Selika Josiah Talbott, Feb 2021 (https://www.forbes.com/sites/selikajosiahtalbott/2021/02/22/are-us-roads-built-for-an-autonmous-vehicle-future/?sh=2dfaa5344874)
- https://www.quora.com/What-is-the-difference-between-transfer-learning-and-federated-machine-learning
- Axios “Automakers are finally starting to share road safety data”, Sudha Jamthe, June 2019,(https://www.axios.com/automakers-are-finally-starting-to-share-road-safety-data-adbcc9f1-efcf-4e07-8a6d-d6b9af9bb185.html)
- Axios, “Automakers are experimenting with blockchain for AVs,” Sudha Jamthe, (https://www.axios.com/automakers-are-experimenting-with-blockchain-for-avs-e439f7ea-3776-415f-b589-00125b04b59e.html)
- CapGemini AI in Automotive report https://www.capgemini.com/wp-content/uploads/2019/03/Ai-in-automotive-research-report.pdf
Author:
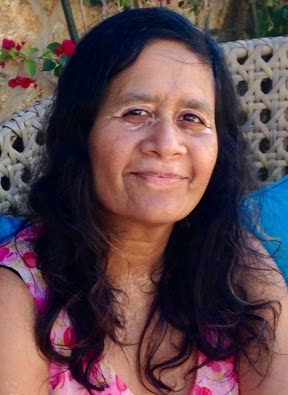
Sudha Jamthe
CEO
IoT Disruptions
Sudha Jamthe is a globally respected Technology Futurist, author of 6 books, speaker and teaches Autonomous Vehicles and AI for business leaders at Stanford Continuing Studies and the Business School of AI. Her research focuses on value creation from data, future of mobility and ethical human centered design of Artificial Intelligence. Ms. Jamthe enjoys mentoring business leaders to bring innovation to their companies and cities.
Published in Telematics Wire