AIPS on predictive models for UBI data:All Roads Do NOT Lead to Rome
“Most insurance carriers don’t know exactly what data builds the best UBI predictive models, and focusing on the wrong data can create misrepresentations of driving behavior and lead to false positives. Unfortunately, you won’t know that you have the wrong data until some years later when you find out that the predictive model you built for your UBI product is not providing the lift that you expected it to provide.”
Jeff Stempora, CEO | Advanced Insurance Products & Services
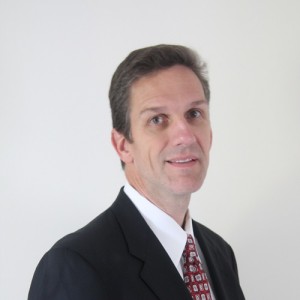
One of the greatest difficulties in developing UBI products that are both profitable and exhibit high levels of pricing sophistication is the collection and meaningful interpretation of the right data. While the insurance industry has always been data-centric, the type of data insurers focus on to achieve higher levels of pricing sophistication has shifted from simple, independent historical and demographic data elements to dynamic, contingent driving performance data from sensors and other new sources. These data elements include speeding, hard braking, time of day, cornering, and more recently, contextual data such as weather, road type, traffic, etc. Unlike their predecessors, these new data types are time series data and therefore require the use of time series analysis or a process to derive a more static parameter that represents the time series (much like a credit score) to be useful. More importantly, the raw data collected is often full of noise and false positives so it needs a substantial amount of cleaning and translation before it can be used.
The challenge that insurance companies face when they start out is that they do not know what specific data or portion of data is truly predictive in nature, and they must collect much more data for a period of years before they can get to the point of identifying exactly what is predictive (the more factors you use in your models, the more data you need to collect for longer periods of time). There are no standard definitions of exactly what constitutes a cornering or acceleration event. Insurance companies that have figured this out are not going to share as this is part of the fundamental secret sauce of usage based insurance and what creates a state of information asymmetry in the industry.
Also, working with this new type of data is a lot like learning a new language; it’s not the individual words that matter but the meaning or ideas that they convey when put together.
Additionally, with such large volumes of data being collected, it is now more likely that insurers will find more spurious correlations within that data that can lead them astray, especially when using existing technology methods to mine the data and build predictive models (Remember Allstate and zodiac signs?). The point is, although there are all kinds of advanced technologies that are now coming to market which propose to solve all of the big data problems, at the end of the day for UBI it comes down to putting in the time to research and develop the interpretation of the data followed by correlations with the right data based on trial and error. Just like learning that new language, it takes time to be able to think and interpret in new ways and to learn all of the grammatical nuances. Based on history and actual experience, this takes about 8 to 10 years to get it right.
Knowing that there is a long and expensive knowledge curve that comes with mastering UBI, is helpful in terms of planning, and if everyone in the industry was just starting out, we could all progress at a moderate pace toward our end goal of creating a profitable and effective UBI product. Unfortunately, the fact is that a few insurers have already put in the time and effort and have developed first versions of UBI scoring models that are at least a little better than credit scoring models, and they are now aggressively moving toward their second versions. So the question for risk management institutions like insurers is, “Are you going to gamble with pursuing your own efforts to create a competitive UBI product while the first movers will be that much further ahead by the time you finish your first product?” Some probably assume that they will be able to catch up just like they did when credit scoring was introduced. What they fail to understand is that this situation is very different from the credit scoring situation. This time the data is not in a standard, mature and widely available form like credit was so it will take much longer to match the capability of an insurer that has UBI scoring already. In the end, many insurers will be forced to outsource their UBI product or purchase packaged solutions that have predictive models incorporated within them.
In my 30 years in the insurance and financial services industry, I can’t remember a time when the information asymmetry was so great and the divide between the have’s and the have not’s was so large, so the odds are not in favor of those who are not in the know.
The “Right” Data
The amount of data is only one issue – using it effectively is another. The fact is most insurance carriers don’t know exactly what data builds the best UBI predictive models, and focusing on the wrong data can create misrepresentations of driving behavior and lead to false positives. Unfortunately, you won’t know that you have the wrong data until some years later when you find out that the predictive model you built for your UBI product is not providing the lift that you expected it to provide.
Let’s look at hard braking, for example. Many insurers are now capturing hard braking events to assess a person’s driving performance. But what exactly constitutes a hard brake? There is no universal definition of what a hard brake is, and specific incidents of hard braking are not equally predictive in nature. A number of vendors provide an arbitrary definition of hard braking in their products, but have they done the statistical analysis to know that that definition is predictive? A vehicle that hits a pot hole or speed bump, for example, can be interpreted as a hard braking event in many of the solutions in the market today. This results in a false positive that will reduce the effectiveness of the UBI predictive models that you develop from that data. The only way to get past this is to do the statistical analysis to find and validate the predictive characteristics of a hard braking event and then collecting only those events that meet that criteria. Arbitrarily defining hard braking events results in sub-optimal predictive models which produce little to no advantage over present day credit scoring models.
Much like the zodiac signs mentioned previously, UBI predictive model development is replete with the types of problems exemplified by the hard braking example. The trouble is that an insurer will collect the wrong data, perform data mining and statistical analysis, will come up with some initial correlations, and will proclaim victory. These lesser correlations occur because of common correlations between the various data elements – all braking events are correlated to UBI predictive braking events, and UBI braking is correlated to losses. You will never discover this shortcoming unless you perform the full analysis of braking events to statistically define the characteristics of a UBI predictive hard braking event or you wait until your competitor has eaten your lunch.
The point is that collecting the right data is critical to developing an effective and profitable UBI product. In fact it is the most critical step after defining a UBI product strategy. Many insurers start with the selection of a telematics device and use the data that it produces to form UBI predictive models. In this situation, insurance companies let the device drive their UBI product strategy. Although this puts those insurers in a common state with other insurers that have chosen the same course, it will not serve as a defense against those insurers that have validated the right data first and have chosen a device which specifically provides that data. The worst part about it is that once an insurer discovers that its predictive models cannot compete with the likes of those that have the right data, they will have to start all over again because YOU CAN’T GO BACK AND COLLECT THE RIGHT DATA FROM THE PAST. The stakes are high and there is much to lose by not pursuing the creation of UBI predictive models that have at least the same predictive power as those of UBI first movers. When it comes to UBI, all roads do not lead to Rome. When you don’t know what you don’t know, you can save yourself a lot of time, money, and frustration by enlisting the assistance of an experienced guide to help you get there.
About the author
Jeff has over 30 years of executive experience in the insurance industry. His prior roles in the industry include: CEO and co-founder, The Evogi Group; EVP and Global Head of Strategy, Citigroup/Travelers; Strategy Officer and Board member, State Farm Mutual Insurance Companies; SVP and Executive Director, Erie Insurance; Chairman, Risk and Security Executive Committee of the Financial Services Roundtable; and CTO, CNA Financial. He also serves as an advisor and board member for several other companies. Jeff has a BSEE in computer design, an MBA in finance, and a Doctor of Management in Organizational Leadership.